Predicting the Future
In 2015 we asked court professionals from around the world to assess the probability that predictive technology would move courts to become preventive rather than reactive. A hallmark of America’s judicial system is that it is both independent and reactive. Citizens bring their disputes to us; we do not go looking for them. Given that, it is not surprising that 468 responses assessed a possible future involving preventive justice with a resounding “no way.”1
And yet looking at new trends in justice processes, it seems as if predicting the future under the banner of crime prevention is increasingly possible. Automated risk-assessment algorithms are becoming more popular with courts around the country. The aim of these algorithms is to give judges another tool to help predict who will return to court for their future proceedings and who will not; who will commit a serious new crime while awaiting their court date and who will abide by their pretrial conditions; and who will turn their life around and who will struggle while released. Using algorithms raises an important question on its own, one that is more critical since it is a component of the larger discussion we are having about the propriety of fines, fees, and bail within the criminal justice system.
Justice Before Algorithms
About 12 million people are booked into jails every year; most are arrested for property, drug, or public-order offenses.2 Over 60 percent of those arrested are racial or ethnic minorities.3 Before risk-assessment algorithms, judges based their release decisions on their instincts. They worked with little more than a bail schedule, an arrest report, a criminal-history rap sheet, possibly a pretrial-release-interview questionnaire, and the few questions the judge could ask the defendant. At the same time, the standard practice for many prosecutors was to oppose almost all bail or release in the name of public safety. Commonly, release hearings (a.k.a. bail hearings) lasted only a few minutes. Anxiety over releasing the “wrong” defendant always simmered just below the surface.4 Even today court calendars are crammed, judges are overworked, court staff are often harassed, and defendants are commonly rushed through almost en masse. Not surprisingly research has shown that high-risk defendants are often released, while low-risk defendants remain in jail.5 We are also aware that all of us, even judges, have within us implicit bias.6
Algorithms: What Are They Looking At?
Crime-prediction tools were first introduced in the 1900s. They explicitly used nationality and race as determining factors.7 When risk-assessment algorithms came along, they provided some degree of cover against the claim of implicit bias by relying on empirical big data and evidence-based modeling. Algorithms could help identify defendants as flight risks more accurately than judges could on their own.8 An array of factors gathered from thousands of defendants allowed algorithms to more accurately predict who is a good release risk, who is a moderate risk, and who is just downright risky. The inventory of potential statistical factors for weighing risk of flight is long and impressive. Below is just a sample:9
- Current charge or charges
- All pending charges
- Outstanding warrants
- Previous law-enforcement contacts (criminal history)
- Arrests
- Convictions
- Convictions specifically for violent crimes
- Previous failures to appear
- Previous incarcerations
- Probation or parole status
- Mental health
- Social contacts (e.g., gang affiliations)
- Residence
- Length
- Own or rent
- Delinquent on payment
- Employment
- Age (Maturity)
- Drug or alcohol history
- Parents’ criminal history
- Working phone
- Education
- Citizenship
- Marital status
They Really Do Help
It is undeniable that risk-assessment algorithms have benefited the courts, the community, and the criminal-justice system. Statistical evidence shows these algorithms reduce the number of pretrial defendants left to languish in jail and have shortened their incarceration.10 They can lower system costs and reduce the number of time-sensitive, high-pressure court hearings. They can also increase a defendant’s ability to keep a family together and keep a job. They enhance community safety in the long run.11
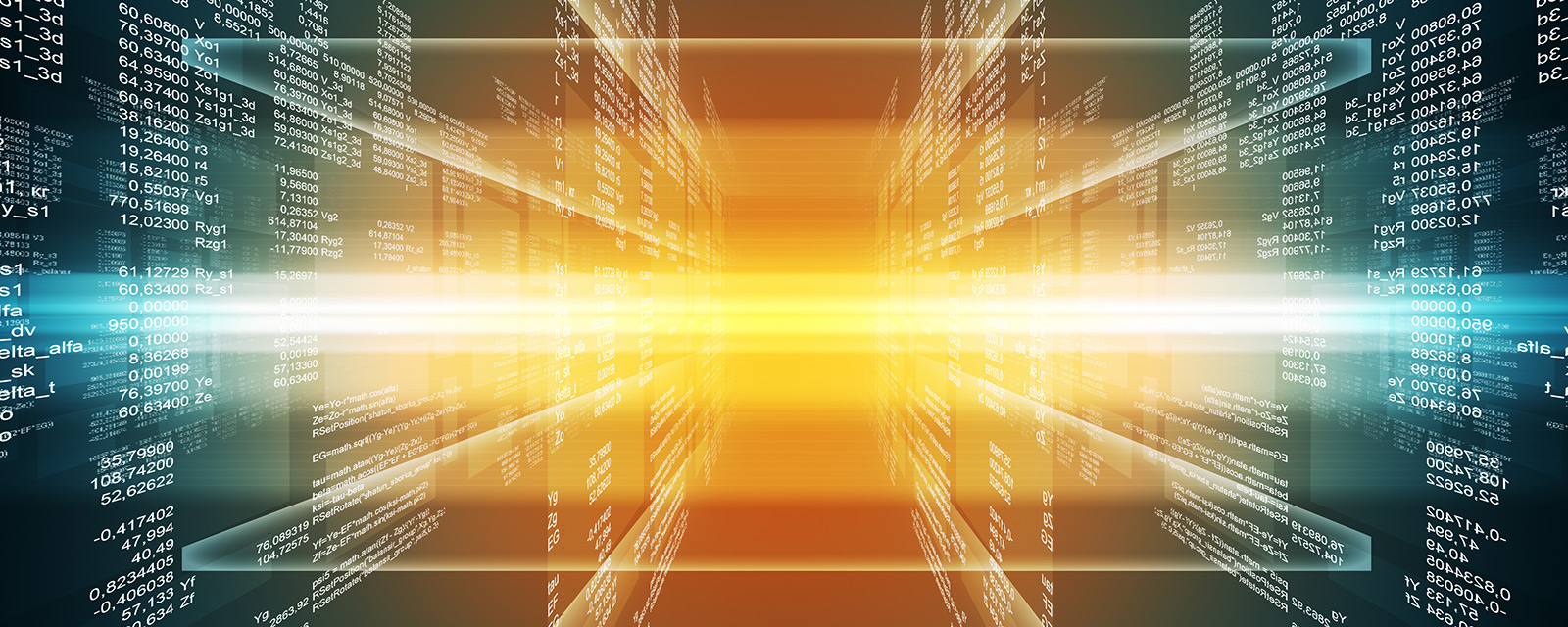
Yet despite these claims of overall effectiveness, many contend that algorithms have systemic bias built directly into their design. They replace the judge’s implicit bias with a form of discrimination based on quantitative data.12
Questions Raised
Given that algorithms improve overall system effectiveness, why not use them everywhere? Should court administrators recommend algorithms to their judges as an important new tool in pretrial release? Or if they are systemically biased, is that bias more pernicious than judges making decisions based on whatever information they have at the time? Can we design out systemic bias?
Predictive Policing
A reliance on big data is an element of the argument that algorithm design holds the potential for systemic bias. Law-enforcement agencies are always looking to maximize effectiveness. By relying on hundreds of thousands of data records, agencies now use big-data-based predictive policing to see where police presence can make the greatest impact. It is no surprise that one of the best predictors of future criminal events is a history of past criminal events. Therefore, more patrols naturally go where past crime has already been high.
Broken Windows
The second question becomes what kind of crime should be tracked? All crime? Just violent crime? Felonies? Misdemeanors? In the last 20 years, an old philosophy has emerged anew: “broken windows.” When it was initiated, the philosophy was widely praised; some studies even celebrated it for reducing New York City’s jail population.13 At its essence, “broken windows” meant if police stopped minor crimes (e.g., the breaking of windows of abandoned buildings), it would eventually reduce serious crime. So for “broken windows” to work, big data needed to track all police contacts. This gave big data even more to work with, since most people booked into jail were there for nonviolent crimes.14 At this point a self-reinforcing negative-feedback loop appeared. If police patrol specific areas more often, people living in those areas will typically have more police contacts and more arrests. This, in turn, leads to more police patrolling of those areas.15
Though not a clear straight line, proxies (e.g., location, previous police contacts, social contacts, etc.) serve to connect race to risk. As an example, although studies have shown roughly the same percentage of drug use in white communities as in minority communities, minority men are arrested at significantly higher rates than white men for drug crimes.16 In some large cities, almost 80 percent of minority men can expect to be arrested and incarcerated at some point in their life.17
Algorithms Ain’t Just for Pretrial Anymore
Algorithms help judges predict the future for pretrial release. They help judges decide who stays in jail and who gets out; who will get a high bail set and who might be released on their own recognizance. Although we are limiting this discussion to the effects of pretrial risk assessment, it is worth noting that these algorithms help predict the future in even more ways. Many states (e.g., Virginia) have created algorithms specifically for sentencing.18 Other states allow judges to the use pretrial risk-assessment scores to assist in sentencing defendants after conviction.19
So What?
America has a whole lot of folks incarcerated and a really large percentage of them are people of color. In less than 30 years, America’s prison population has swelled from 300,000 to over 2 million.20 We now have the highest incarceration rate of any nation in the world, beating out Russia.21 The cost of keeping that many people incarcerated has been estimated at $14 billion a year.22
Over the last 40 years, a term to describe this phenomenon has become popular: mass incarceration. With the extraordinarily high number of minorities imprisoned, America now has a higher percentage of minorities behind bars than South Africa did at the height of apartheid.23 Interestingly, the term itself sparks fierce controversy and raw feelings. Some, including a prominent district attorney, have called the term “mass incarceration” an urban myth perpetuated by both liberals and conservatives.24
Can We Take Bias Out of Algorithms?
Some contend that algorithms are the harbinger of a new paradigm. They are the forerunner of artificial intelligence; they are systems that “learn.” But what does it mean to “learn”? Algorithms can be described as logical quantitative models (albeit complex models) designed to produce results. Various inputs are given values (or weights), then the algorithm produces an output risk score based on calculating the combinations of the weighted inputs. The newest, most sophisticated algorithms modify the weights based on a continual stream of newly acquired information from the inputs. For example, if inputs start showing more of a connection between pretrial drug use and court-hearing failures to appear, the algorithm “learns” to increase the input weight for pretrial defendant drug use. Subsequent defendants who then admit to drug use during a pretrial interview receive a higher risk-assessment score, and a resulting lower chance of being released.25
But can algorithms incorporate entirely new inputs? The answer: Not without human help. We will use a classic baseball Moneyball example. In 1998 the Tampa Bay Rays baseball team decided against signing Albert Pujols, a decision the team’s management probably regrets to this day since Pujols went on to become one of the greatest players of all time. Let us suppose Tampa Bay was using algorithms to sign players back then (something most baseball teams use now). After seeing how well Pujols played for the St. Louis Cardinals, Tampa Bay management would probably go back, figure out what factor they were missing, and add it to their algorithm. That way they would not miss that next great player that comes along. An algorithm cannot figure this out on its own.26
In fact, it appears that risk-assessment algorithms suffer from that self-fulfilling feedback loop mentioned earlier. To return to Albert Pujols, he was free to sign with another team, which he did. He then helped the St. Louis Cardinals to two World Series. Defendants with high risk-assessment scores, however, are not free to just walk out of jail. They are more likely to remain in custody, more likely to lose their jobs, more likely to have their families abandon them, and more likely to lose their worldly belongings. They are more likely to face a powerful incentive to plead guilty just to get out of jail. Pleading guilty to a felony makes it almost impossible to get a steady job as defendants must report their felony conviction on most job applications. The lack of employment opportunity drives many to a life of crime and, thus, it negatively affirms what the algorithm predicted all along. If a court uses an algorithm to help with sentencing, this could result in longer prison sentences and an even greater likelihood of the defendant recidivating.27
It might be best to describe the Pujols example not as “learning,” but as “discovering.” Without creative human intervention, algorithms cannot incorporate new inputs. Risk-assessment algorithms cannot account for a man who turned his life around, was chatting with his neighbor at 1:00 a.m. after a long second shift at work, and was arrested in a sweep for blocking pedestrian traffic.28
We acknowledge the counterargument, that algorithms do not replace judicial decisions, they merely complement those decisions with empirical data. One article clearly states this admonition—it is important to remember that algorithms are not intended to replace the independent discretion of judges.29 True enough, but with a typical bail hearing lasting only a minute or two and given the confidence that data-driven assessments provide, the incentive to override the risk assessment cannot be strong.30 Additionally many risk-assessment algorithms are proprietary, meaning that the organizations that developed them have a vested interest in not sharing the internal logic, the inputs, or the respective weights of those inputs. They have an interest in proving only that the algorithms work. Due to this natural competition, there needs to be unbiased monitoring and review of results.
As court administrators, we are in a unique position regarding the use of risk-assessment algorithms. We must evaluate the efficacy of these algorithms and make some sort of a recommendation to the court. Do we recommend the concept of algorithms because, although flawed, they are better than judges making decisions without this supplemental data? Do we recommend only nonproprietary, open-sourced algorithms so we are allowed to “peek under the hood”? Do we recommend not using algorithms in favor of previous methods of decision making relied on for years? Do we lobby for algorithms to be modified and, if so, who would do it? How would we even know if the modifications have improved things?
Court Leaders Weigh In
We asked Penny Stinson, president of the National Association of Pretrial Service Agencies; Dale Allen, chief probation officer for Athens-Clarke County Probation Services in Georgia; Greg Lambard, chief probation officer for the New Jersey Superior Court in Burlington Vicinage, Mount Holly, New Jersey; Craig Levine, director of policy reform for the Bronx Defenders in the Bronx, New York; and Sarah Couture, paralegal assistant for the 13th Judicial Circuit in Tampa, Florida to comment on pretrial risk-assessment algorithms.
Questions
Does your court’s pretrial agency currently use an automated risk-assessment algorithm?
Pretrial risk-assessment algorithms, although popular, are not by any means universal. Greg Lambard said that that his court uses an algorithm. Sarah Couture responded that her court does not. Dale Allen replied that his court does not use an algorithm for misdemeanor probation, though they are currently close to completing a technology project that will offer judges an “offender information dashboard.” The dashboard will give a broad picture of the offender, with components determining both successful and unsuccessful completion of probation.
Do judges make more informed decisions when they have access to algorithms like these?
Penny, Greg, Dale, and Sarah all said that algorithms provide judges with more information and can lead to better decisions. Penny commented that actuarial pretrial risk assessments have been tested to show that, while they are not flawless, they produce improved outcomes over the use of judicial decision making alone. Actuarial risk-and-needs assessments have repeatedly outperformed professional judgment alone across multiple meta-analyses, different populations of justice-involved individuals, and varied measures of recidivism.31
Penny warned that assessments are not meant to replace judicial discretion, but rather to inform not only judges, but also prosecutors, defense attorneys, and pretrial personnel. “Additionally, these assessments can be used to help craft the least onerous (as required by law) conditions of release. Given that assessments of risk and need are used for critical decisions within the criminal justice field, including allocation of valuable resources, and we know the harm involved in incarcerating defendants for even the briefest periods of time, it is vital that professionals rely on the most accurate procedure available.”32
Greg and Dale both wholeheartedly agreed. Greg noted, “Before [these algorithms] judges never had an objective assessment of risk to fail to appear and to recidivate prior to disposition.” Dale said that his experience has been that judges appreciate as much data about an individual as we can provide. “They still make the decision, but I sincerely believe that more data that can be provided accurately and concisely is very valuable.”
Sarah agreed but was slightly more cautious. “Yes, it gives them more detailed information than they might have access to if not using a risk-assessment tool, but it also does not remove the potential for racial and ethnic biases on the part of the judges. It gives judges an additional information set that they can utilize in making their decisions while taking evidence and other factors into consideration that the algorithm does not in their decision-making process.”
Penny also advised that the algorithms should not be used in any trial-based or posttrial decisions. “No tool predicts how an individual person will behave; they show only how that person compares to others who are similarly situated and provides evidence of how those others behaved in the past.” This is a critical admonition since we have seen evidence of courts in a few states doing exactly that: using pretrial assessments in sentencing.
Craig Levine objected strongly, arguing that risk-assessment instruments should have no role in bail determination and no place in “bail reform” conversations. The concern is that the excitement over risk-assessment instruments (RAIs)33 in bail determination—and in the broader discussion of “predictive analytics” arising in multiple contexts—could lead to the contrary result of imposing greater impositions on defendants’ liberty. “It is important to focus on what is or should be the problem being addressed: over-incarceration, particularly of people of color. This is the issue that largely brought RAIs into the broader criminal justice reform conversation and should be the policy lodestar.” Craig posits that any proposed bail reform must be judged, first and foremost, on its ability to reduce the numbers of people detained pretrial, reduce racial bias, and reduce bias based on wealth. “Risk assessments should play no role in these conversations, which should focus on securing a wide array of changes in policing, court processing, and due process.”
Are risk-assessment algorithms biased and how would we even know?
Craig is convinced that algorithms’ pernicious effects are inevitable and implicate fundamental questions of racial and ethnic equality, as well as due process of law. RAIs bring a tantalizing illusion of scientific objectivity, but not its reality. First, RAIs are only as good as the data that go into them. If the input (arrest and conviction data, for example) is, as in New York, tainted by structural racial bias, the output (risk determinations) will reflect the same bias. Indeed, studies have shown that “bias in criminal risk scores is mathematically inevitable.”34
Research has found that racial disparities are inevitable. Craig pointed out, “A risk score… could either be equally predictive or equally wrong for all races—but not both. There is thus a very real danger, indeed a high likelihood, that RAIs and the appeal of ‘objective risk scores’ would silently codify racial disparities in bail determinations under a veneer of scientific rigor.”
Sarah conjectured that, to a certain extent, risk-assessment algorithms, being data driven, are not an individualized decision. They do not take into account each individual’s story. “The algorithm could be biased based on gender and race if those factors are included in the risk assessment.” She pointed out that an algorithm could, over time, be determined as biased or not through analysis of data and the scores generated for certain groups of individuals. For instance, are there disparities among different groups based on race and gender when looking at similar offenses?
Penny responded that the meta-analysis of research indicates that risk-and-needs assessments are not inherently biased in and of themselves. The use of criminal history is definitely found to be a moderating variable that explains the relationship between race and elevated risk levels.35
“[W]e caution that the utilization of criminal histories is indicative of bias in other decision points within the system. Even recognizing that the era of community policing and ‘broken windows’ may have disproportionally targeted communities of color, the poor, and the disenfranchised, it is not believed that the elimination of risk-and-needs assessments would result in a system that is less biased. The bottom line is that objective assessments have continually proven to be a better predictor of risk than the use of professional judgment alone.”
Multiple studies, including one completed by Marie Van Nostrand, on this topic have indicated that the design-validation processes of actuarial pretrial risk-assessment instruments virtually eliminate racial bias. As a result, the Pretrial Justice Institute advises, “A common misperception of these tools is that they rely heavily on defendants’ prior arrests to determine the pretrial risk score, thereby discriminating against people of color who, for a variety of reasons, may have higher rates of previous justice system involvement. This is not the case. While no pretrial assessment tool can erase racial and ethnic disparities elsewhere in the criminal justice system, evidence-based assessments are designed to be race- and gender-neutral and regular testing can ensure they continue to have no disparate impact.”36
Dale and Greg also think algorithms are less biased than judicial decision making alone. Greg said, “The public-safety assessment in New Jersey was first validated against New Jersey data and the researcher found it to be gender and race neutral.” Dale reminds us that algorithms only report data. “The interpretation of that data may be biased. I believe human interaction and review, especially in early stages and follow up thereafter, will assist in keeping the system normed and ‘honest.’”
Are court administrators obligated to advocate for improving risk-assessment algorithms?
Craig said that no one thinks someone should be in jail because they are poor; however, algorithms are not the only, best, or even a good way to reduce incarceration levels. Algorithms are outcome-neutral, so they can be used to increase pretrial detention as easily as to decrease it. A recent report by Human Rights Watch notes that at least one jurisdiction that uses the popular Laura and John Arnold Foundation’s Public Safety Assessment has seen its pretrial release rate decline, but the rate at which defendants plead guilty at first appearance double.37
Craig reminded us that the definition of “risk” is ultimately a policy question. “Risk-assessment tools may be adapted in the future to fill or empty jails, depending on the direction of the political winds. For example, policymakers in New Jersey have demanded alterations to the state’s new, much-touted bail reform measures to require pretrial detention of people charged with certain crimes.”38
Sarah and Greg both said that they believe that we, as court administrators, must continue to advocate for improving these algorithms. “As more data becomes available, as instruments are improved over the years, we need to ensure we do not have outdated algorithms. Realizing that these are predictive models, there will always be a need to improve upon them.” Sarah said that the COSCA policy papers on evidence-based pretrial release, and the National Task Force on Fines, Fees and Bail Practices, are proof of our need to never be satisfied, but to continue promoting increasing accuracy.
Dale said that court administrators, probation chiefs, and any other staff that directly support the judicial system should advocate for any system that assists judges in making these important decisions on a daily basis.
Penny said that while studies show that that risk-and-needs assessments can make predictions without inherent racial or ethnic bias, we know that no pretrial assessment tool can erase racial and ethnic disparities elsewhere in the criminal justice system. First, any risk-and-needs assessment must be validated on the individual jurisdiction’s target population. “It is important that courts understand that pretrial risk-assessment tools are based on an analysis of a court’s closed cases. Many ‘off-the-shelf’ tools have been developed using national data (taken from the federal system). Consequently, it is imperative that courts continue to measure these assessments against local data to ensure that the questions and their weighting are accurately predicting pretrial success and failure.”
It must also be remembered that while risk-and-needs assessments can help inform frontend and backend decisions geared toward reducing risk and keeping appropriate individuals in the community, these assessments can be misused.39 Jurisdictions deciding to use risk assessment need to choose an appropriate algorithm, devote the time and resources necessary to validating it on the population for which it will be used, and commit to periodically revalidating it to ensure continued efficacy.40
As with any data, we all know the validity of data is only as good as the accuracy of the data being collected and entered. Court administrators should never be fooled into thinking that just because the instrument has a minimal number of questions that everyone administering it is asking it in a way so that the information elicited is valid. Penny added, “As a result, courts must ensure frequent initial and periodic trainings offered to support the validity of collected information and the scoring results.”41
Penny suggested that it is imperative that courts embrace the use of validated assessments. “To accomplish this, court administrators are in a unique position to champion this initiative. They can ensure that judicial officers, court staff, prosecuting attorneys, defense attorneys, defendants, and the public are educated on the importance of using these assessments. They can also help change the culture by emphasizing the importance of frontend decision making and by supporting what they are teaching with what they are doing (e.g., experienced judges and magistrates at initial appearance, non-case-specific outreach to their local newspaper editorial board and civic groups, funding the frontend appropriately).”
Finally, as a country that has experienced mass incarceration, Penny reminded us of the harm inflicted by incarcerating people even for brief periods of time. “Court administrators are a crucial piece of the equation in ensuring their courts abide by the tenants of the Trial Court Performance Standards. They are the individuals tasked with the responsibility of ensuring the court adheres to fundamental principles of fairness and can ensure their courts embrace a culture of adherence to research and evidence-based practices.”
Takeaways
In reviewing the respondents’ opinions, observations, and feedback, we are left with three “takeaways.”
Algorithms are an improvement, but there is still work to be done.
Although not everyone agrees that algorithms are an improvement, they appear to reduce the number of incarcerated individuals at pretrial and increase the predictive accuracy of who should remain in jail and who should not. The National Institute of Corrections reports that the new recidivism predictors generally have a 73 percent accuracy rate, which is a significant improvement over a 55 percent accuracy rate when largely using judicial discretion alone. This means, however, that 27 percent of the time we still get it wrong.
There is still much work to be done, and court administrators must be at the forefront pressing for more progress. Once we have celebrated the superior accuracy of algorithms, the philosophy of continuous improvement calls for us to start the cycle again and attempt to improve predictive accuracy by exploring ever more subtle factors, but ones that could move the needle to 75 or even 80 percent accuracy.
Let us also remember that judges can and do override the algorithms. Court administrators must advocate for more detailed data on when judges base their decision on the algorithms and when they override it by asking questions such as, “When they do override the algorithm, what is their reasoning? Could that reasoning eventually become quantifiable?”
Data are needed regarding the length of time defendants remained in jail when denied release. What happened to their jobs? What happened to their families? Did they eventually choose to plead guilty just to be released? When defendants are released either based on the algorithm or against it, did they return to court? Did they commit a new crime? Did they commit a violent crime?
Systemic bias may not be primarily racial or ethnic; it may be socioeconomic.
Many who write on this topic (including in this article) do not make a clear distinction between racial ethnic bias and socioeconomic class bias, so the two seem to conflate. This might be a mistake. Even though the two components significantly overlap, they are different and probably need independent analysis. Clearly, many of the algorithms have gone to great lengths to eliminate racial and ethnic bias; much less has been written about how they treat socioeconomic class. As there is significant overlap, it is unclear if a new approach will also reduce racial disparities.42
We must be proactive.
Court administrators tend to be a somewhat conservative lot in decision-making style, often deferring action until an issue is fully joined. This style may not be workable in the future. A recent news story concerning new medications marketed to drug courts nationally has shown that outside groups could, and often do, simply go around court administrators in advocating for new policies.43
This article mentioned that the New Jersey legislature altered the pretrial risk-assessment weight factor for gun possession during a crime. Although it is understandable to politically alter an input weight like gun possession, algorithms are objective, quantitative probability models. By way of analogy, a tenet of probability theory is that a repeatedly flipped coin (over the long run), will come up heads as often as it comes up tails. An outside body changing an input weight based on politics, rather than an objective probability of returning to court or committing a new crime, is akin to declaring that a coin will come up heads 20 percent more often simply because the group wants it to happen that way, not because it actually will.
When we see political tinkering, we must call out that an algorithm so altered is no longer a quantitatively predictive model, but a political model hiding under the guise of empirical data. Tinkering with the weights will eventually discredit the entire process and compel judges to return to the days of using only judicial decision making. We must be advocates because, more than likely, no one is going to go out of their way to ask our opinion.
We deeply appreciate Sarah Couture, Penny Stinson, Dale Allen, Craig Levine, and Greg Lambard for their insights on the new aspects of this critical and controversial criminal justice innovation. Have a question or comment? Email us at futureofcourts@gmail.com.
ABOUT THE AUTHORS
Peter C. Kiefer is the civil court administrator for Maricopa Superior Court in Phoenix, Arizona. He has been questioning ethics for Court Manager since 1994. Phillip Knox is principal consultant of KSA Consulting, LLC.
NOTES
* Thanks to Nicole Garcia and T. J. BeMent for their editing and research on this article. This article was previously published on the National Center for State Courts’ Trends in State Courts website (October 2017).
- Phillip Knox and Peter C. Kiefer, Future of the Courts—2015 Survey.
- Laura and John Arnold Foundation, “Developing a National Model for Pretrial Risk Assessment,” LAJF Research Summary (November 2013).
- Cynthia A. Mamalian, “The State of the Science of Pretrial Risk Assessment,” report, Pretrial Justice Institute, March 2011.
- Id.
- Laura and John Arnold Foundation, supra n. 2.
- Oren Gazal-Ayal and Raanan Sulitzean-Kenan, “Let My People Go: Ethnic In-Group Bias in Judicial Decisions—Evidence from a Randomized Natural Experiment,” Journal of Empirical Legal Studies 7 (2010): 403-28.
- Jon Schuppe, “Post Bail: America’s Justice System Runs on the Exchange of Money for Freedom. Some Say That’s Unfair. But Can Data Fix It?” NBC News, August 22, 2017.
- Tom Simonite, “How to Upgrade Judges with Machine Learning,” MIT Technology Review (March 6, 2017).
- Pretrial Justice Institute, “Pretrial Risk Assessment: Science Provides Guidance on Assessing Defendants,” Issue Brief (May 2015).
- John DeRosier, “Jail Population Down 19 Percent in N.J. Since New Bail Reform Law,” Atlantic City Press, July 17, 2017.
- Angèle Christin, Alex Rosenblat, and Danah Boyd, “Courts and Predictive Algorithms,” Data and Society (October 27, 2015).
- Julia Angwin, Jeff Larson, Surya Mattu, and Lauren Kirchner, “Machine Bias: There’s Software Used Across the County to Predict Future Criminals. And It’s Biased Against Blacks,” Pro Publica (May 23, 2016).
- James Austin and Michael P. Jacobson, How New York City Reduced Mass Incarceration: A Model for Change? (New York: Vera Institute for Justice, 2013).
- Laura and John Arnold Foundation, supra n. 2.
- Michelle Alexander, The New Jim Crow: Mass Incarceration in the Age of Colorblindness (New York: New Press, 2010).
- Cathy O’Neil, Weapons of Math Destruction: How Big Data Increases Inequality and Threatens Democracy (New York: Crown Publishing, 2016).
- Alexander, supra n. 15.
- Cara Thompson, “Using Risk and Need Assessments to Enhance Outcomes and Reduce Disparities in the Criminal Justice System,” Myth and Facts series, National Institute of Corrections, March 2017.
- Jason Tashea, “Courts Are Using AI to Sentence Criminals. That Must Stop Now,” Wired (April 17, 2017).
- Alexander, supra n. 15.
- “United States Incarceration Rate,” Wikipedia (last edited October 3, 2017).
- Schuppe, supra n. 7.
- Alexander, supra n. 15.
- Robert J. Smith, “Red Justice in a Blue State,” Slate (January 13, 2017), describing Josh Marquis, district attorney for Clatsop County and past president for the Oregon District Attorneys’ Association.
- O’Neil, supra n. 16.
- Id.
- Alexander, supra n. 15.
- Matt Taibbi, The Divide: American Injustice in the Age of the Wealth Gap (New York: Spiegel and Grau, 2014).
- Laura and John Arnold Foundation, supra n. 2.
- Christin, Rosenblat, and Boyd, supra n. 11.
- Thompson, supra n. 18.
- Id.
- The terms “risk-assessment instrument (RAI)” and “risk-assessment algorithm” are synonymous.
- Julia Angwin and Jeff Larson, “Bias in Criminal Risk Scores Is Mathematically Inevitable, Researchers Say,” ProPublica (December 30, 2016); see also Angwin et al., supra n. 12.
- J. L. Skeem and C. T. Lowenkamp, “Risk, Race, and Recidivism: Predictive Bias and Disparate Impact,” manuscript under review, 2016; later published in Criminology 54 (2016): 680-712.
- Pretrial Justice Institute, “Race and Pretrial Risk Assessment,” n.d.
- Human Rights Watch, “Not in It for Justice: How California’s Pretrial Detention and Bail System Unfairly Punishes Poor People” (April 11, 2017): 99-100.
- See S. P. Sullivan, “AG Wants Automatic Jail Time for Gun Cases Under N.J. Bail Reform,” NJ.com (April 24, 2017).
- Pretrial Justice Institute, supra n. 36.
- Thompson, supra n. 18.
- Pretrial Justice Institute, supra n. 36.
- Schuppe, supra n. 7.
- Jake Harper, “To Grow Market Share, a Drug Maker Pitches Its Product to Judges,” National Public Radio (August 3, 2017).